LLM
large language models
Created:
See also generative-ai page. and smol-llm, transformer-math, LlamaIndex, RAG, and local-llm, llm-embedding, AI SaaS, LLM Training, Reward Models
Introductory Materials
Generative AI exists because of the transformer – A visual story from Financial Times; Sept 2023.
Large language models, explained with a minimum of math and jargon
Study Guides
- The Novice’s LLM Training Guide; copy
- Normcore LLM Reads by Vicki Boykis
- A Hackers’ Guide to Language Models - YouTube by jeremy-howard
- Transformer Math 101 | EleutherAI Blog
Blogs
- Lil’Log
Hi, this is Lilian. I’m documenting my learning notes in this blog. Other than writing a ML blog, I’m leading Applied Research at OpenAI on the side.
- Finbarr Timbers – eg: Five years of GPT progress
Papers
- Sparks of Artificial General Intelligence: Early experiments with GPT-4. (2023) PDF – Bubeck, Sébastien, Varun Chandrasekaran, Ronen Eldan, Johannes Gehrke, Eric Horvitz, Ece Kamar, Peter Lee, et al. “Sparks of Artificial General Intelligence: Early experiments with GPT-4,” 2023.
- SeamlessM4T—Massively Multilingual & Multimodal Machine Translation | Meta AI Research – Barrault, Loïc, Yu-An Chung, Mariano Cora Meglioli, David Dale, Ning Dong, Paul-Ambroise Duquenne, Hady Elsahar, et al. “SeamlessM4T-Massively multilingual & multimodal machine translation,” 2023. https://arxiv.org/abs/2308.11596.
- Brown, Tom B., Benjamin Mann, Nick Ryder, Melanie Subbiah, Jared Kaplan, Prafulla Dhariwal, Arvind Neelakantan, et al. “Language models are few-shot learners,” 2020. https://arxiv.org/abs/2005.14165.
Videos
An observation on Generalization - YouTube by Ilya Sutskever (OpenAI); Aug 14, 2023.
- Supervised Learning - precise mathematical condition under which learning should succeed, which is - Low training error + more training data than “degrees of freedom” = low test error
Prompt Engineering
- ChatGPT Prompt Engineering for Developers - DeepLearning.AI
- Prompt Engineering | Lil’Log – “This post only focuses on prompt engineering for autoregressive language models, so nothing with Cloze tests, image generation or multimodality models.”
- Controllable Neural Text Generation | Lil’Log – “How to steer a powerful unconditioned language model? In this post, we will delve into several approaches for controlled content generation with an unconditioned langage model. For example, if we plan to use LM to generate reading materials for kids, we would like to guide the output stories to be safe, educational and easily understood by children.”
- Replacing my best friends with an LLM trained on 500,000 group chat messages
- microsoft/guidance:
A guidance language for controlling large language models.
Guidance programs allow you to interleave generation, prompting, and logical control into a single continuous flow matching how the language model actually processes the text.
Models
See this page - Models Table – Dr Alan D. Thompson – Life Architect for a visual representation of models, and a table of various attributes of models.
Stuff you can run on your computer
How is
LLaMa.cpp possible? how can we run
llama.cpp
on
local machines when the expectation is that large models need expensive
GPUS (eg: A100) to run
Introducing
Code Llama, a state-of-the-art large language model for coding
Code Llama is a code-specialized version of Llama 2 that was created by
further training Llama 2 on its code-specific datasets, sampling more
data from that same dataset for longer. Essentially, Code Llama features
enhanced coding capabilities, built on top of Llama 2. It can generate
code, and natural language about code, from both code and natural
language prompts (e.g., “Write me a function that outputs the fibonacci
sequence.”) It can also be used for code completion and debugging. It
supports many of the most popular languages being used today, including
Python, C++, Java, PHP, Typescript (Javascript), C#, and Bash.
Ask HN: Cheapest way to run local LLMs? | Hacker News
See also Perplexity Labs where they have multiple models to try from
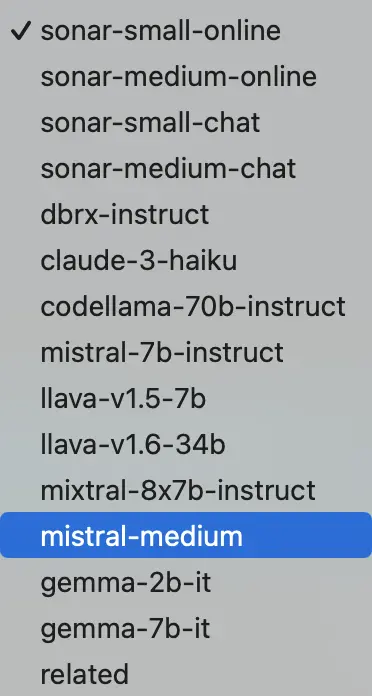
LLMs in your language
All languages are NOT created (tokenized) equal
Small Language Models
- Eldan, Ronen, and Yuanzhi Li. “TinyStories: How Small Can Language Models Be and Still Speak Coherent English?” 2023.
Using LLMs
- A Simple but Powerful Method to Analyze Online PDFs with Bing Chat - AI Demos
- What’s new in Llama 2 & how to run it locally - AGI Sphere; Aug 2023.
- Fine-tune your own Llama 2 to replace GPT-3.5/4 | Hacker News > Fine-tuning has one huge advantage though: it is far more effective at guiding a model’s behavior than prompting, so you can often get away with a much smaller model. That gets you faster responses and lower inference costs. A fine-tuned Llama 7B model is 50x cheaper than GPT-3.5 on a per-token basis, and for many use cases can produce results that are as good or better! > For example, classifying the 2M recipes at https://huggingface.co/datasets/corbt/all-recipes with GPT-4 would cost $23k. Even with GPT-3.5 it would cost over $1k. The model we fine-tuned performs similarly to GPT-4 and costs just $19 to run over the entire dataset. > OpenBMB/ToolBench: An open platform for training, serving, and evaluating large language model for tool learning.
Frameworks
Haystack | Haystack
Open-source LLM framework to build production-ready applications
.
> Use the latest LLMs: hosted models by OpenAI or Cohere, open-source
LLMs, or other pre-trained models > All tooling in one place:
preprocessing, pipelines, agents & tools, prompts, evaluation and
finetuning > Choose your favorite database: Elasticsearch,
OpenSearch, Weaviate, Pinecone, Qdrant, Milvus and more > Scale to
millions of documents: use Haystack’s proven retrieval architecture >
Compare it to LangChainAI
GPT4All A free-to-use,
locally running, privacy-aware chatbot. No GPU or internet
required.
an AI proxy that lets you use a variety of providers (OpenAI, Anthropic, LLaMa2, Mistral, and others) behind a single interface w/ caching & API key management.
MLC LLM Machine Learning
Compilation for Large Language Models (MLC LLM) is a high-performance
universal deployment solution that allows native deployment of any large
language models with native APIs with compiler acceleration. The mission
of this project is to enable everyone to develop, optimize and deploy AI
models natively on everyone’s devices with ML compilation
techniques.
Multimodal Learning
todo
Security
OWASP | Top 10 for Large Language Models
Operational Issues
- Why won’t Llama13B fit on my 4090_.pptx - Google Slides by Mark Saroufim
GGUF
GGUF and GGML are file formats used for storing models for inference, especially in the context of language models like GPT
Benchmarking LLMs
LLM Benchmark Report for: NousResearch/Redmond-Puffin-13B
Linkdump
2023
- Shoggoth is a peer-to-peer, anonymous network for publishing and distributing open-source code, Machine Learning models, datasets, and research papers.
- What We Know About LLMs (Primer) #primer
- A comprehensive guide to running Llama 2 locally - Replicate – Replicate; Jul 22, 2023.
- PaLM2 Technical Report #pdf #google
- dalai – Run LLaMA and Alpaca on your computer. ??
- ggerganov/llama.cpp: Port of Facebook’s LLaMA model in C/C++
- LlamaIndex - (GPT Index) is a project that provides a central interface to connect your LLM’s with external data. see https://twitter.com/gpt_index
- LLM Introduction: Learn Language Models
- Announcing OpenFlamingo: An open-source framework for training vision-language models with in-context learning | LAION
- become a 1000x engineer or die tryin’
- Simon Willison: LLMs on personal devices Series.
- How You Can Install A ChatGPT-like Personal AI On Your Own Computer And Run It With No Internet.
- How to run your own LLM (GPT); Apr 2023.
- modal-labs/quillman
A complete chat app that transcribes audio in real-time, streams back a response from a language model, and synthesizes this response as natural-sounding speech. This repo is meant to serve as a starting point for your own language model-based apps, as well as a playground for experimentation.
- A brief history of LLaMA models - AGI Sphere
- See LangChainAI
- ray-project/llm-numbers: Numbers every LLM developer should know; via HN
- Beginner’s guide to Llama models - AGI Sphere; Aug 2023.
- The Mathematics of Training LLMs — with Quentin Anthony of Eleuther AI
- Google Gemini Eats The World – Gemini Smashes GPT-4 By 5X, The GPU-Poors; Aug 2023.
- fast.ai - Can LLMs learn from a single example?
2024
Survey of Open source repos – What I learned from looking at 900 most popular open source AI tools (via)
Allen-Zhu, Zeyuan, and Yuanzhi Li. “Physics of language models: Part 3.3, knowledge capacity scaling laws,” 2024. https://arxiv.org/abs/2404.05405.
You, Keen, Haotian Zhang, Eldon Schoop, Floris Weers, Amanda Swearngin, Jeffrey Nichols, Yinfei Yang, and Zhe Gan. “Ferret-UI: Grounded mobile UI understanding with multimodal LLMs,” 2024. https://arxiv.org/abs/2404.05719.
About BERT – Geiping, Jonas, and Tom Goldstein. “Cramming: Training a language model on a single GPU in one day,” 2022. https://arxiv.org/abs/2212.14034. – via
Ma, Xuezhe, Xiaomeng Yang, Wenhan Xiong, Beidi Chen, Lili Yu, Hao Zhang, Jonathan May, Luke Zettlemoyer, Omer Levy, and Chunting Zhou. “Megalodon: Efficient LLM pretraining and inference with unlimited context length,” 2024. https://arxiv.org/abs/2404.08801.; github repo (the repo link on paper wasn’t working as of 2024-04-17).
“phi-3-mini, a 3.8 billion parameter language model trained on 3.3 trillion tokens, whose overall performance, as measured by both academic benchmarks and internal testing, rivals that of models such as Mixtral 8x7B and GPT-3.5 (e.g., phi-3-mini achieves 69% on MMLU and 8.38 on MT-bench), despite being small enough to be deployed on a phone.” Abdin, Marah, Sam Ade Jacobs, Ammar Ahmad Awan, Jyoti Aneja, Ahmed Awadallah, Hany Awadalla, Nguyen Bach, et al. “Phi-3 technical report: A highly capable language model locally on your phone,” 2024. https://arxiv.org/abs/2404.14219. (No code, or model was announced with the paper.)
Liu, Ziming, Yixuan Wang, Sachin Vaidya, Fabian Ruehle, James Halverson, Marin Soljačić, Thomas Y. Hou, and Max Tegmark. “KAN: Kolmogorov-arnold networks,” 2024. https://arxiv.org/abs/2404.19756.
Lin, Yiming, Madelon Hulsebos, Ruiying Ma, Shreya Shankar, Sepanta Zeigham, Aditya G. Parameswaran, and Eugene Wu. “Towards accurate and efficient document analytics with large language models,” 2024. https://arxiv.org/abs/2405.04674. “Unstructured data formats account for over 80% of the data currently stored, and extracting value from such formats remains a considerable challenge…. ZenDB efficiently extracts semantic hierarchical structures from such templatized documents, and introduces a novel query engine that leverages these structures for accurate and cost-effective query execution. Users can impose a schema on their documents, and query it, all via SQL. Extensive experiments on three real-world document collections demonstrate ZenDB’s benefits, achieving up to 30% cost savings compared to LLM-based baselines, while maintaining or improving accuracy, and surpassing RAG-based baselines by up to 61% in precision and 80% in recall, at a marginally higher cost.”
References
- Meta Reference - letsbuild.ai
- Keeping up with AGI reading list by cto_junior.
- LLM Course by mlabonne
Multimodal Learning Multi Agent Frameworks AI Agent Framework