Reward Models
Created:
Lambert, Nathan, Valentina Pyatkin, Jacob Morrison, LJ Miranda, Bill Yuchen Lin, Khyathi Chandu, Nouha Dziri, et al. “RewardBench: Evaluating reward models for language modeling,” 2024. https://arxiv.org/abs/2403.13787.

With RewardBench, we:
- Release a common framework for evaluating the many different architectures of reward models, along with tools for visualization, training, and other analysis. We also release all data used in the evaluation, composed of text-score pairs for all inputs, to enable further data analysis on the properties of reward models.
- Illustrate the differences between DPO and classifier-based reward models across a variety of datasets. DPO models, while more plentiful due to the method’s simplicity, fail to generalize to popular preference data test sets and present a higher variance in performance.
- Chart the landscape of current state-of-the-art reward models. We showcase the scaling laws, the propensity to refuse (or not), the reasoning capabilities, and more for popular RMs.
- Show the limitations of existing preference data test sets for evaluating these models, showcasing common pitfalls of RMs on subtle, but challenging instruction pairs (e.g. intentionally modified rejected responses, which superficially look high quality but answer the wrong prompt).
Yuan, Weizhe, Richard Yuanzhe Pang, Kyunghyun Cho, Xian Li, Sainbayar Sukhbaatar, Jing Xu, and Jason Weston. “Self-rewarding language models,” 2024. https://arxiv.org/abs/2401.10020.
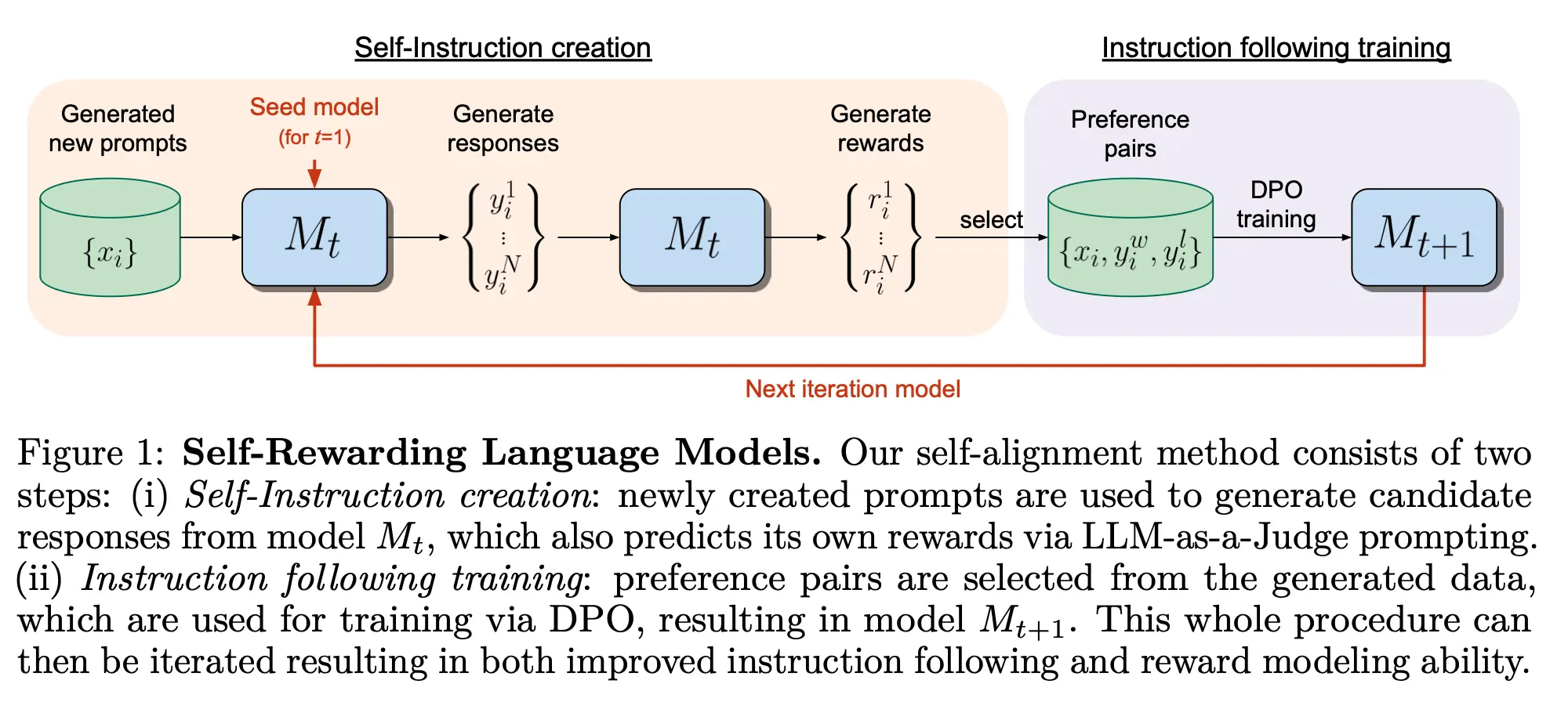
Current approaches commonly train reward models from human preferences, which may then be bottlenecked by human performance level, and secondly these separate frozen reward models cannot then learn to improve during LLM training. In this work, we study Self-Rewarding Language Models, where the language model itself is used via LLM-as-a-Judge prompting to provide its own rewards during training. We show that during Iterative DPO training that not only does instruction following ability improve, but also the ability to provide high-quality rewards to itself.
Self Play Fine Tuning (SPIN)
According to Das, Sayantan. “Model Alignment Process,” 2024., (creator of premai, which is also a good overview of RHLF (Reinforcement Learning from Human Feedback), DPO (Direct Preference Optimization), IPO (Identify Preference Optimization), the propose Self-Play Fine-Tuning (SPIN) is a method designed to enhance the capabilities of Large Language Models (LLMs) through a unique self-improvement process that doesn’t rely on additional human-annotated data – Chen, Zixiang, Yihe Deng, Huizhuo Yuan, Kaixuan Ji, and Quanquan Gu. “Self-play fine-tuning converts weak language models to strong language models,” 2024. https://arxiv.org/abs/2401.01335.